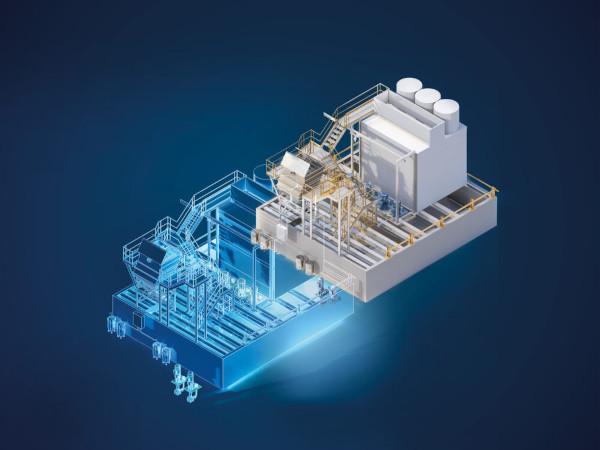
Date: 6 June 2024
When analysing errors in manufacturing processes, using AI and more specifically Deep Learning technologies makes for rapid and precise analysis and interpretation of ever more complex, extensive datasets. The objective: fewer defects and less downtime, producing the same product in less time while using less energy and resources. The theme “AI in machine technology” is also an important topic at glasstec 2024, the leading trade fair not only for glass producers but also for the machine manufacturers involved. On behalf of Messe Düsseldorf the author spoke to VDMA’s Glass Technology Forum and renowned industry players in the run-up to the fair.
The VDMA also looks at the topic of Artificial Intelligence as part of innovation management. Gesine Bergmann (Head of the VDMA Glass Technology Forum) reports: “It is the adaptability of machinery and equipment builders that drives innovations and allows our industry to remain competitive. Here joint research for our member companies plays an essential role.” Artificial Intelligence generally denotes the ability of a machine or computer system to perform tasks that would usually require human intelligence – such as learning and problem solving, language recognition and reproduction, image recognition and in future maybe also something like “intuition” from experience. How to possibly attribute this type of intelligence to a machine was already researched by the British mathematician and IT specialist Alan Turing back in the 1950s. His “Turing Test” aims to assess the ability of a machine to show human-like behaviour or to demonstrate human-like intelligence. The basic idea: man and machine interact based on text from separate rooms, and the human does not know whether they are communicating with a machine or another human being. If the human cannot reliably decide, the Turing Test is considered as passed. This test still serves as factual criterion to assess human-like interaction of machines today and as a basis for numerous discussions about the development of AI systems and also their ethical implications.
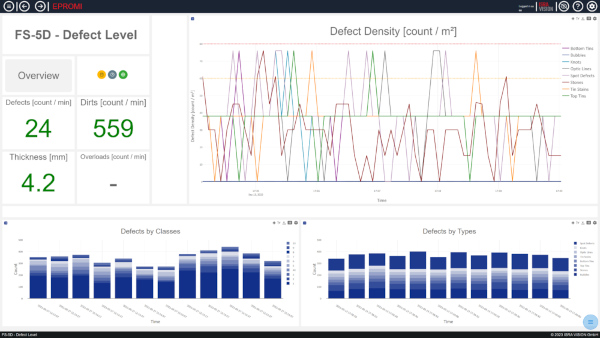
Chart: ISRA VISION GmbH
Use of AI in the flat glass industry
Modern AI algorithms are often based on machine learning or so-called Deep Learning. Machine learning as a subsegment of Artificial Intelligence focuses on the development of algorithms, which can learn from data without having been explicitly programmed for it. They identify patterns of, and relations between data making forecasts or taking decisions based on this. Deep Learning, in turn, is a method of machine learning that uses multi-layered, deep neuronal networks. These networks are highly performant in data processing as they detect complex structures in vast data volumes and are capable of extracting abstract features from this. This is precisely the “high potential that the flat glass industry can still tap into,” reports Peter Seidl, Head of Product Management at machinery producer Grenzebach. This technology innovator serves the flat glass industry with tailor-made automation solutions for the production and processing of industrial float glass.
Seidl: “For many of the processes running in those highly automated factories experience shows nothing is more important than having an experienced operator in all important positions from the melting unit through to monitoring all processes in the IT centres. Here, the industry and modern factories are already very advanced and the efficiency of glass production and the resulting glass quality using conventional means have almost reached the limits of optimisation. The use of Deep Learning AI still offers plenty of potential especially for defect detection – and assessment.” Those looking at the progress made by the flat glass industry will be surprised: “While some decades ago the cameras used in production were only able to tell good from bad glass sheets, today’s systems are so highly performant that a multitude of data is made available for defect analysis permanently”. Seidl explains: “Thanks to image analysis and information gathered by sensors Deep Learning models can identify the smallest defects, complete defect patterns or irregularities in the manufacturing process quickly and precisely, recognise patterns in data and infer potential root causes from these. AI can then translate these assessments into recommended actions for the respective operator to optimise production “on the fly”. AI cannot replace experienced members of staff but it can provide and support them with increasingly better information.”
Likewise, the complexity of the complete glass journey from the hot to the cold end of production is an ideal field of application for AI: batch, glass melt, heating, cooling, sizing – a complex interplay of many line segments and hundreds of parameters and states which often mutually influence each other. This is difficult to understand and manage even for the most experienced operators. Malfunctions occur abruptly or already become visible as trends. AI-assisted systems, however, can forecast them based on smallest indicators and prevent them if they arise by means of early alerts. Seidl explains: “This does presuppose that the knowledge about all process steps is digitalised and pre-processed to train the AI so it can identify correlations at the end of the day. In this process it is constantly learning about thickness changes, climate conditions, all influence factors – sooner or later the AI will be capable of capturing and assessing all factors.”
ISRA Vision GmbH also already uses AI systems. The company offers inspection solutions for the glass industry – which by their own accounts detect 100% of defects in float glass and processed products, at full production speed and with reliable classification in real time. ISRA systems monitor the complete process to optimise the glass yield in a resource and energy-saving manner. One concrete example of the use of AI is the EPROMI solution, as Florian Sterzing, R&D Team Leader Data Analytics at ISRA Surface Vision knows. “This solution makes the potential hidden in production data visible and helps to infer decisions along the value chain. To this end, it collects data from inspection systems and other sources available, processes them and visualises them in intuitive dashboards. The most important and most heatedly disputed development area currently is predictive analytics where AI-assisted systems analyse historical trends, identify patterns and anticipate bottlenecks and inefficiencies, thereby prompting continuous process improvements.
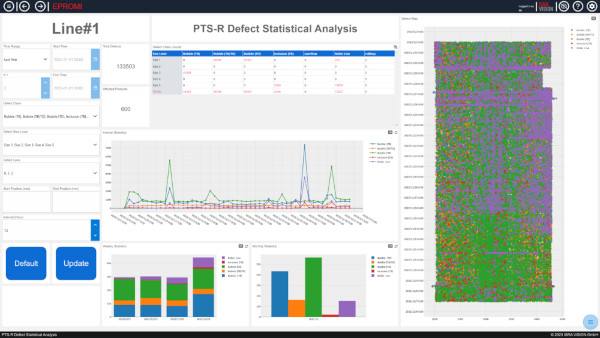
Chart: ISRA VISION GmbH
Initially, these systems learn by given data and then also from each experience acquired. Specialised decision-making AIs can be trained for every application, every production area that generates data. This helps to cut maintenance times, avoid downtimes and, hence, also reduce CO2 emissions: the same product, made in less time with less resources and energy used.”
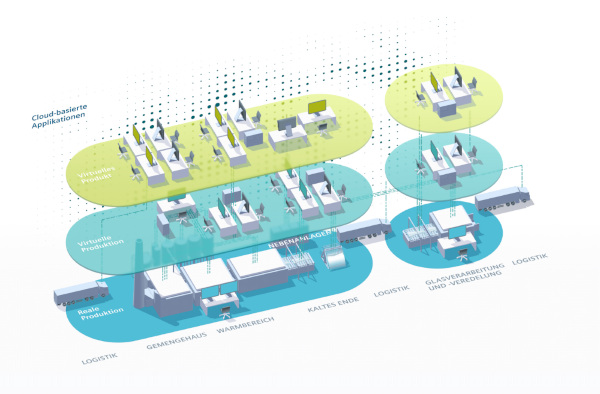
Legend: Cloud-based Applications,
Virtual Product,
Virtual Production,
Real Production,
Peripheral Equipment,
Logistics Doghouse Hot End Cold End Logistics Glass Processing/ Glass Finishing Logistics
Chart: Siemens AG
Learning with the digital production twin
Tobias Wachtmann, Head of Vertical Glass & Solar Siemens Digital Industries, deals with digitalisation along the entire value chain of glass production. Asked about the possibilities for Artificial Intelligence in this field, he came back with a counter question: “For what objective? Decarbonisation? Saving resources? Improving working conditions? Increasing energy efficiency?” Many Siemens solutions also found in float glass production, already harness Artificial Intelligence, embedded in systems and services, often in cooperation with partners such as Grenzebach. According to Wachtmann one of the issues is: “Linking the different systems with each other: data from the charge, the melt and from the complete glass ‘journey’ from hot to cold end production processes. The objective here continues to be a stable production process with the highest yield while identifying and realising potential for further optimisation.” This is why Wachtmann regards digital twins as a smart long-term solution for testing and optimising AI solutions: “An exact digital twin of the complete production lines and conditions can allow us to simulate, calculate and repeatedly optimise solutions with AI virtually. The insights and approaches found can then be applied to reality, in order to continuously reduce rejects or optimise energy consumption. On this basis, even machines can be initially built virtually and the AI can be trained for a wide variety of models.”
Ethical implications of disruptive technologies
Every disruptive technology comes with ethical implications: railways, automobiles, the Internet, mobile communication – wherever new technologies emerge there is always also uncertainty as to whether familiar structures will fall by the wayside. It therefore seems essential that AI systems are developed and used in a fair, transparent and ethically responsible manner while also gauging the implications for the world of work at an early stage, with a view to reshaping it with farsightedness and care in the interest of man. You can look forward to this year’s glasstec, where the topic of Artificial Intelligence will also be discussed as one of the Hot Topics on the stages of the glasstec conference.
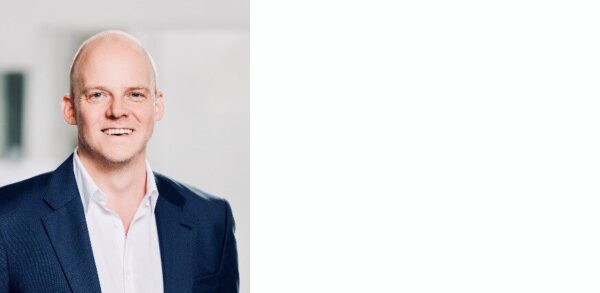
Marc Everling studied media education at the Technical University Brunswick, worked as a consultant in PR and marketing agencies for 14 years, and as Head of Marketing at one of the global flat glass players for six years. In February 2021 he founded his networking agency specialised in communications consulting and press liaison for construction material producers, initiatives, associations, trade fairs and architects which work and produce sustainably in the interests of the ecological transformation of the construction sector. Photo: Marc Everling Nachhaltige Kommunikation
Main photo: A digital twin allows cullet water systems, for example, to be commissioned virtually, operators to be trained comprehensively prior to real operation and automation solutions to be extensively tested on the basis of Simatic PCS 7.
Graph: Siemens AG/PVAG
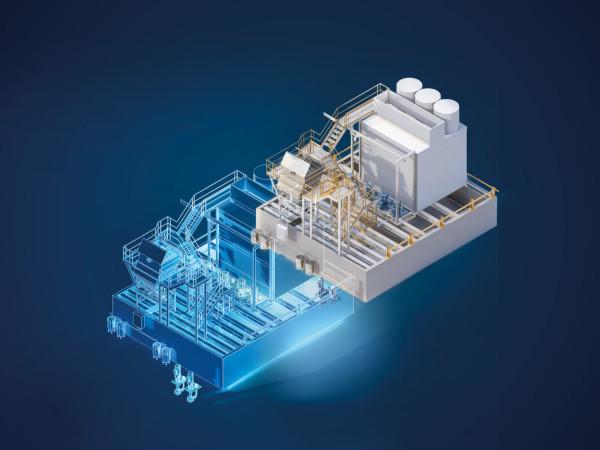

Add new comment